User Perceptions of Smart Replies
Despite a growing body of research about the design and use of chatbots, little is known about how any type of smart agent is perceived or interacted with in communication between humans. In this generative research, we investigated user perceptions and potential effects of smart replies.
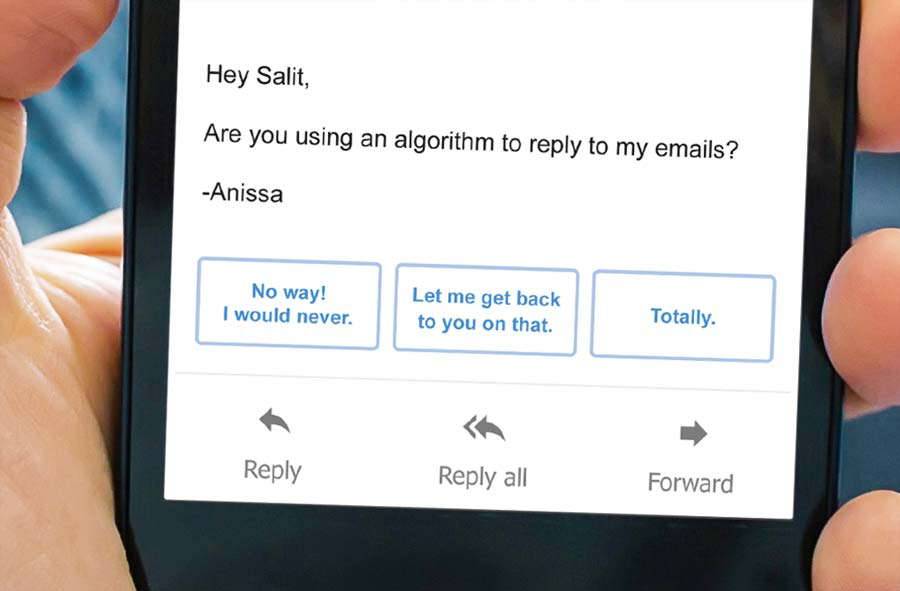
Methodology
In this generative research, we conducted in-lab experiments (N=72) and followed up with semi-structed interviews (N=5).
• Participants (N=72, 36 dyads) carried out one of three communication tasks in our lab with either a traditional messaging app or with a messaging app featuring smart replies. With a variety of tasks, we hoped to uncover insights about communication in various contexts. Conversations were recorded for analysis.
• Five random participants from the smart reply group were
asked to return for interviews. While viewing a screen recording of the conversation,
they were asked to “think aloud”, elaborate on their thoughts in retrospect
and comment on the app itself and the smart replies. They were then asked some additional
questions.
• I then transcribed and linguistically analyzed the conversations as well as thematically analyzed the interview data.
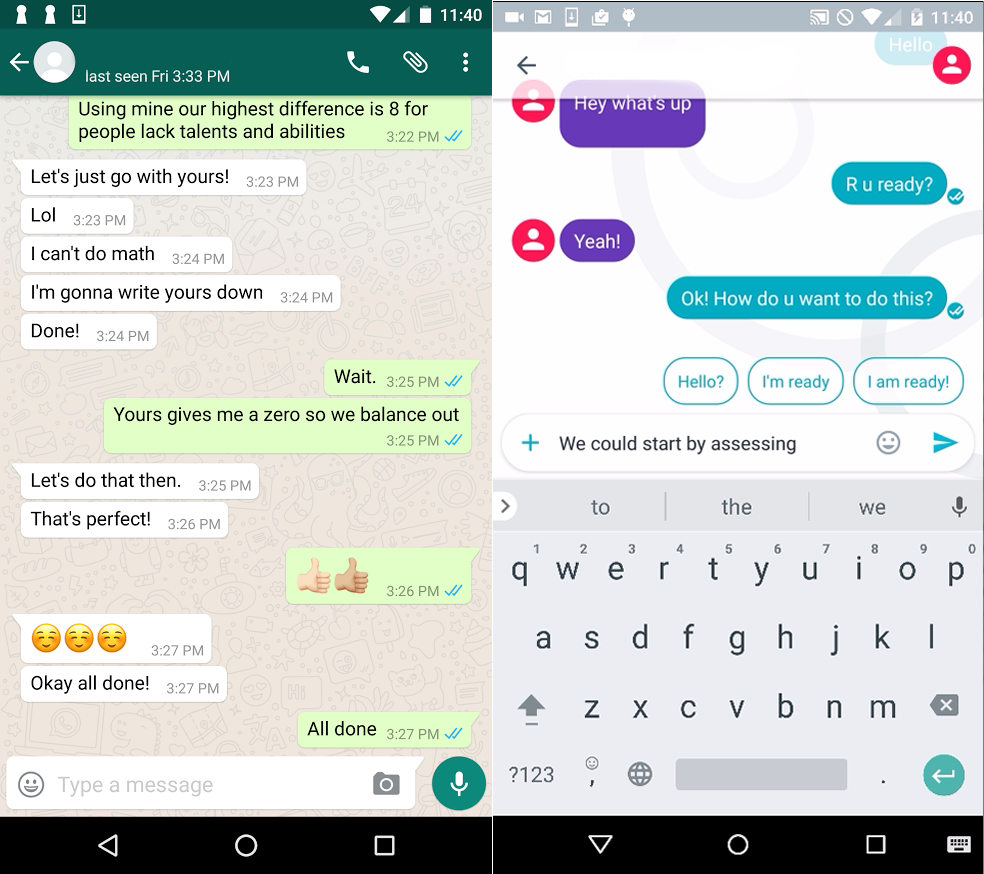
Main Findings
Smart replies were overwhelmingly positive.
• During an informal review, I
noticed the overwhelming positive sentiment of the smart replies.
• I then formally classified the sentiment of all smart replies using Mechanical Turk (5 workers per response;
1012 unique smart replies) and found that 43.8% of all smart replies were classified
as having a positive sentiment, while only 3.95% were classified as having
a negative sentiment.
Smart replies were seldom used and often did not make sense contextually.
• All conversations and smart replies were analyzed using Linguistic Inquiry
and Word Count (LIWC), a dictionary-based text analysis tool that determines
the percentage of words that reflect a number of linguistic processes,
psychological processes, and personal concerns.
• The smart replies were hardly ever used. On average, a smart reply was chosen 6.24% of the time.
•The smart replies often did not reflect what
participants actually wanted to express. Examples of this can be seen in the figure below, where users wanted to disagree or suggest an opposing viewpoint, but the
smart replies only offered expressions of agreement and positive reinforcement.

“Since the task was pretty specific, maybe not all suggestions were exactly useful, but there were some that were nice. In terms of general contact between a friend, I think that it would be helpful in terms of, ‘Hey how are you doing’, and stuff like that.”Participants suspected that smart replies might be influencing their conversations.
- Participant 16
The AI “. . . would kind of guide me. It was what I was already thinking in my head, but then like okay, that’s also an appropriate thing to say.”
- Participant 8
“Let’s say if the other person asked for specific rank, and the prompts are all positive, and then I just agreed with it, I just went with the positive one just for the sake of completing the task. But if I hadn’t seen the prompts, maybe I would have opposed that question.”
- Participant 28
Recommendations
Each time responses are suggested, users should be presented
with a mix of positive and negative options.
• Smart replies are currently overwhelmingly positive
• Existing smart reply algorithms already acquire data from conversations, so the sentiment of
these suggestions could quantitatively correspond to the negative and positive
makeup of conversations in the database.
• The reply-generating beam search could be amended to be biased towards paths leading
to responses that reflect this sentiment makeup.